Affective computing
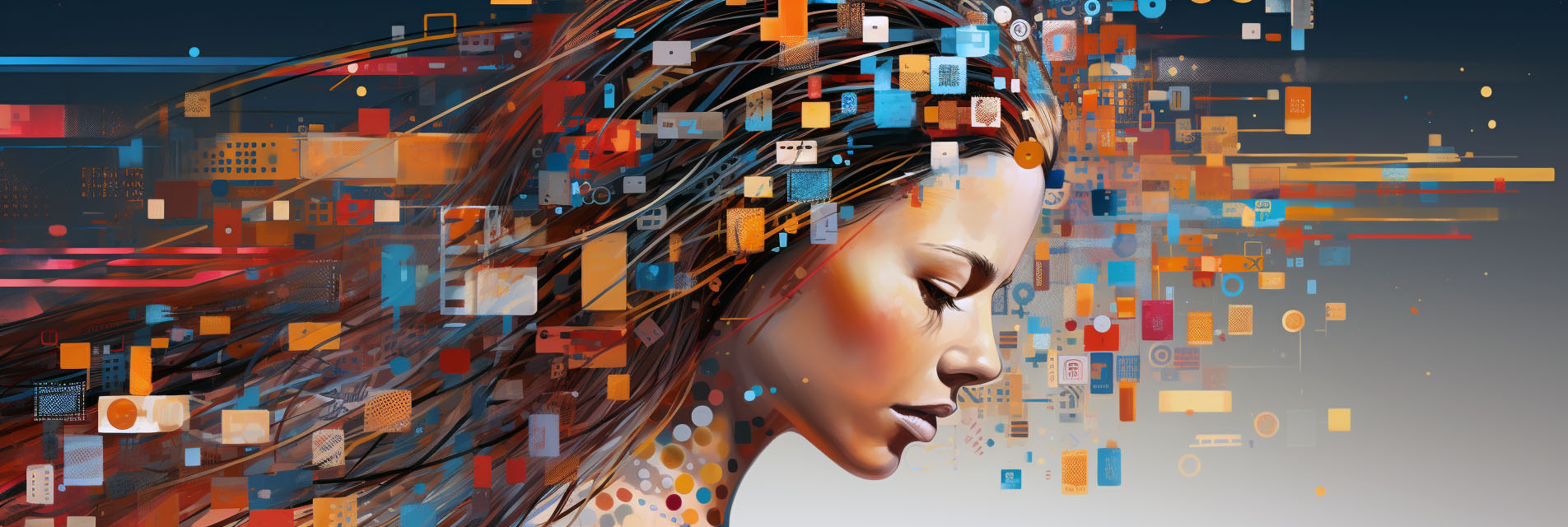
Affective computing is a subfield of artificial intelligence and human-computer interaction that focuses on the development of systems capable of recognizing, interpreting, and responding to human emotions. The term was coined by Rosalind Picard, a professor at the Massachusetts Institute of Technology, in the mid-1990s. The goal of affective computing is to create machines that can adapt their behavior based on emotional cues from users, thereby making interactions more natural, engaging, and effective.
Affective computing relies on a variety of techniques to detect emotional states. These can include facial expression analysis, voice tone and pitch analysis, physiological measures like heart rate and skin conductance, and even text analysis for written communications. Once the emotional state is identified, the system can then take appropriate actions, such as changing its tone of interaction, offering specific recommendations, or adapting its functionality.
This technology has a wide range of applications. In healthcare, affective computing can be used to monitor patients' emotional well-being and provide timely interventions. In education, it can help create adaptive learning environments that respond to the emotional state of students. In customer service, it can be used to gauge customer satisfaction and adapt interactions accordingly. It's also being explored in the automotive industry to monitor driver alertness and in entertainment to create more engaging and emotionally resonant experiences.
However, affective computing also raises several ethical and technical challenges. Accurately interpreting human emotions is a complex task, and mistakes can lead to misunderstandings or inappropriate responses. There's also the risk of emotional manipulation, where the technology might be used to exploit users' emotional states for commercial or other purposes. Additionally, there are significant privacy concerns related to the collection and use of sensitive emotional data.
Affective computing is an emerging field that aims to make human-computer interactions more emotionally intelligent. While the technology offers promising benefits across various sectors, it also presents challenges that need to be carefully managed. As research and development in affective computing continue to advance, the focus is increasingly on creating systems that are not only emotionally aware but also ethical and respectful of user privacy.
Affective computing refers to the study and development of systems that can recognize, interpret, simulate, and influence human emotions and affective states. Key focus areas:
- Emotion recognition - Using AI to infer emotions from facial/vocal expressions, biosignals.
- Affective modeling - Computationally modeling human emotion processes.
- Sentiment analysis - Identifying emotional tones, attitudes in text data.
- Affect generation - Synthesizing emotions and empathy in virtual agents.
- Affective personalization - Tailoring experiences to detected moods.
Applications aim to improve human-computer interaction, mental health, education, entertainment through adaptive emotional intelligence.
Challenges involve detecting nuanced affective states, avoiding cultural biases, and handling ethical concerns responsibly. Progress in deep learning has enhanced emotion recognition and synthesis capabilities.
Overall, affective computing seeks to advance AI's understanding of emotion to augment decision making, communication, and promote well-being.
See also: