Dependence
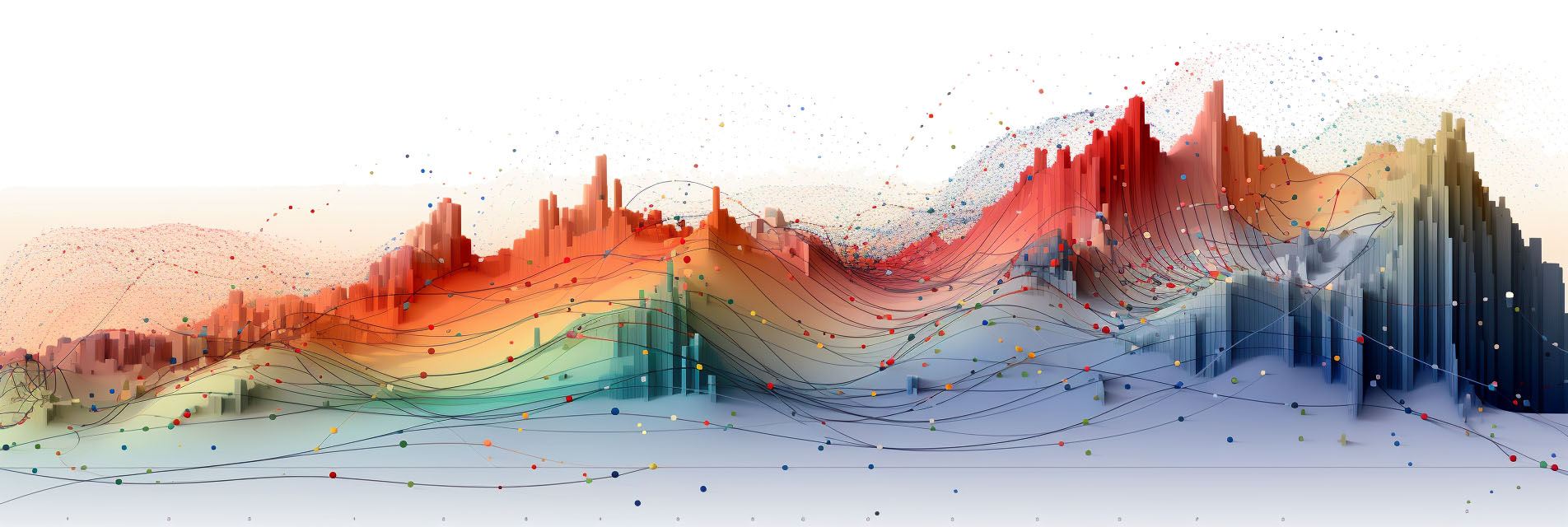
Dependence in statistics refers to any statistical relationship between two or more variables or sets of data. Unlike correlation, which specifically measures the strength and direction of a linear relationship between two variables, dependence encompasses a broader range of relationships, including nonlinear, non-monotonic, and complex multi-variable interactions. In other words, if knowing the value of one variable gives you information about the likely value of another variable, those variables are said to be dependent.
Dependence can manifest in various forms and can be measured using different techniques, depending on the nature of the variables and the relationship between them. For example, mutual information is a measure of dependence that captures the amount of information one variable provides about another, regardless of the type of relationship. Chi-square tests can be used to measure dependence between categorical variables. In finance, copulas are often used to understand the dependence structure between different financial assets.
Understanding dependence is crucial in a wide range of applications. In healthcare, for instance, understanding how different medical variables are dependent on each other can help in diagnosis and treatment planning. In machine learning, understanding feature dependence is important for feature selection and model interpretability.
However, measuring dependence can be challenging. Unlike correlation, which is relatively straightforward to compute, some measures of dependence can be computationally intensive or require large sample sizes for accurate estimation. Additionally, like correlation, measures of dependence are descriptive and do not, by themselves, indicate causality. Establishing a causal relationship between variables generally requires controlled, experimental studies.
In summary, dependence is a broader concept than correlation and refers to any statistical relationship between two or more variables. It can take on various forms, including linear, nonlinear, and complex interactions, and can be measured using a variety of techniques. While understanding dependence is crucial in many fields, it comes with its own set of challenges, including computational complexity and the need for large datasets.