Generative model
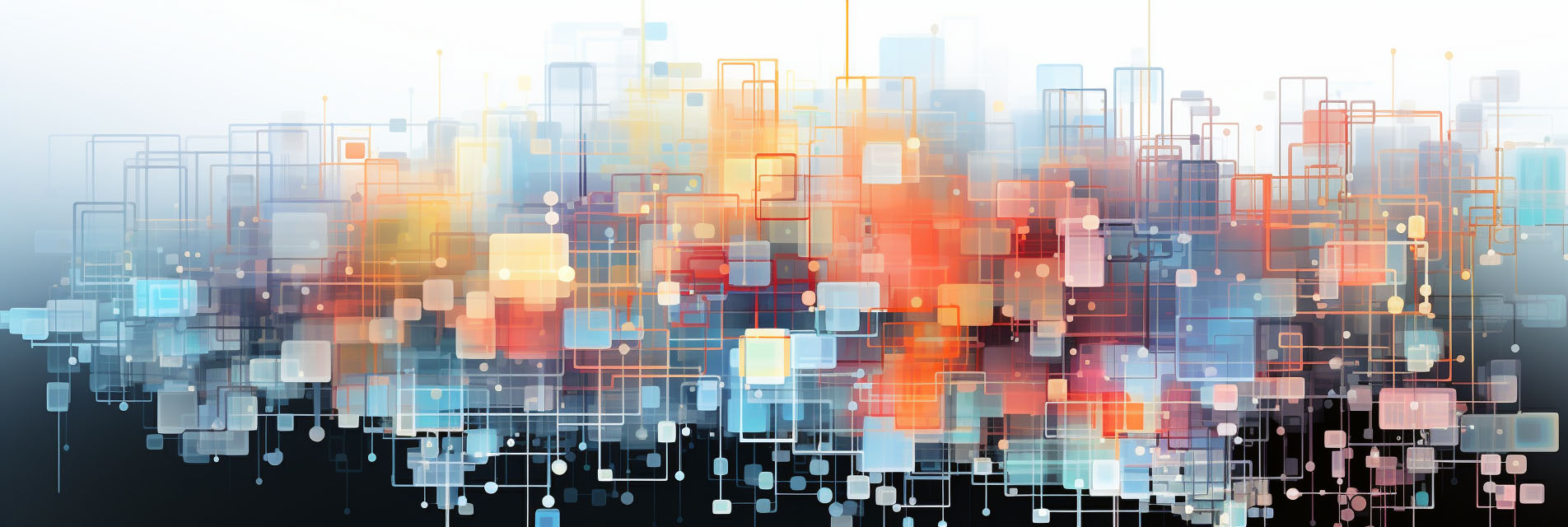
A generative model is a type of machine learning model that is able to generate new data samples from a learned probability distribution. Generative models are useful for tasks like generating synthetic images, audio, video, and text. The key property of generative models is that they do not require large labeled training sets. Instead, they discover patterns and variations within unlabeled training data.
Some common categories of generative models include:
- Generative adversarial network (GANs) - Two neural nets contest with each other to generate realistic outputs
- Variational autoencoder (VAEs) - Learn latent representations and generate by sampling from latent space
- Diffusion model - Gradually denoise and add structure to random noise
- Flow-based model - Invertibly transform simple noise to complex data
- Autoregressive model - Generate data sequentially one part at a time
Unlike discriminative models which classify inputs, generative models allow sampling new data points and exploring the potential space of outputs. They have enabled major advances in AI-generated art, audio, video, and language. Challenges include training instability and controlling the outputs. Overall, generative modeling is a key area of machine learning with many real-world applications.
See also: