John Hopfield
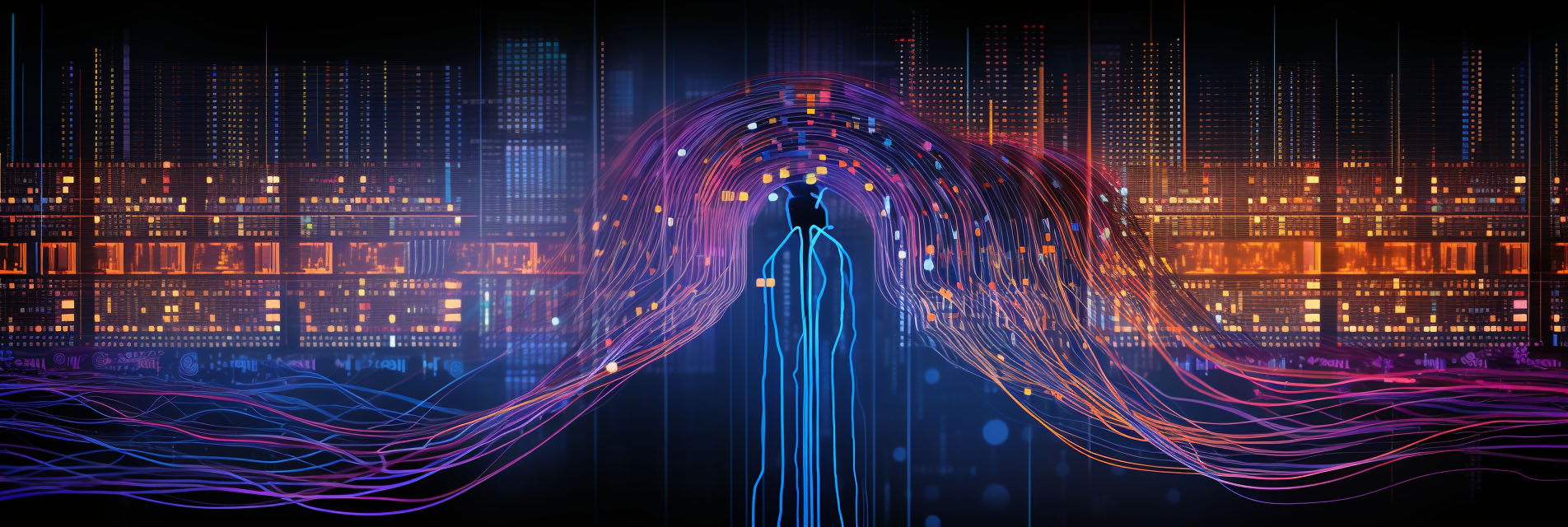
John Hopfield is an eminent American physicist and pioneer in the field of neural networks and machine learning. He made seminal contributions to the hopfield network, a type of recurrent artificial neural network that serves as content-addressable memory system.
After completing his PhD in physics from Stanford University in the 1960s, John Hopfield began conducting research on modeling nerve conduction using statistical mechanics. In 1982, he proposed the concept of the Hopfield network that used an energy minimization framework to store memory patterns as stable states of the network. It used bidirectional connections and threshold activation with nodes that both store information and process it. This provided a model for how human memory may work.
Hopfield Networks have the capacity to retrieve full patterns from incomplete information, acting as associative memory. This content-addressable memory where learnt memories can be accessed without knowing their storage location or address, mimics aspects of mammalian cognitive processes. The recurrent connectivity allows temporal sequences to be stored and high noise tolerance. The Hopfield model created new intersections between physics, computer science and neurobiology.
John Hopfield made other noted contributions like developing the technique of optimal reconstruction from inaccurate data. He introduced the use of random projection matrices for dimensionality reduction. Over his esteemed career, he has held academic positions at Caltech, Princeton, MIT and has received numerous prestigious awards recognizing his groundbreaking work. Even well into his 70s, John Hopfield continues to push research frontiers with his recent focus on understanding how brains work through modeling, algorithms and their computational capabilities.