Memristor
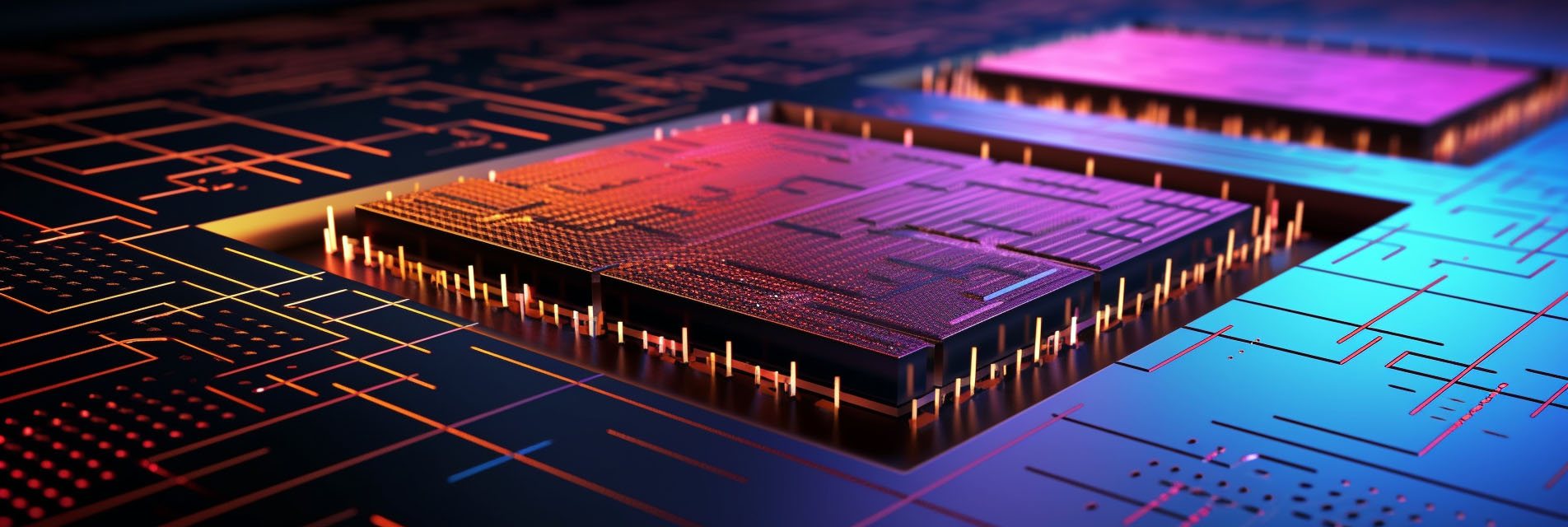
A memristor is a non-linear two-terminal electrical component that relates the integral of electric current (i) to the integral of voltage (v) across the component. The term "memristor" was coined by Leon Chua in 1971, who mathematically derived it as the fourth fundamental circuit element alongside the resistor, capacitor, and inductor.
The concept of the memristor was first introduced by Leon Chua, an electrical engineer and computer scientist, in a seminal paper published in 1971. The paper, titled "Memristor—The missing circuit element," was published in IEEE Transactions on Circuit Theory. In this paper, Chua provided a mathematical foundation for the memristor, describing it as the fourth basic circuit element alongside resistors, capacitors, and inductors.
Chua's work was initially theoretical, aiming to fill a gap in the existing framework of electrical circuit theory. He observed that while there were three basic passive circuit elements to relate the four fundamental circuit variables—current (i), voltage (v), charge (q), and magnetic flux (φ)—there was a missing element that would relate charge and magnetic flux. This led him to propose the memristor, a term derived from "memory" and "resistor."
The memristor was unique in that it provided a relationship between the time integrals of current and voltage, effectively "remembering" its state even when electrical power was removed. However, despite the theoretical foundation laid by Chua, a practical memristor device would not be realized until decades later.
Since its discovery, the memristor has been the subject of extensive research and development. It has applications in various fields such as computer memory, neuromorphic computing, and signal processing.
Memristors have several unique characteristics that set them apart from other electrical components:
- Non-linearity - The relationship between current and voltage is non-linear.
- Memory - Memristors "remember" their last state even when power is removed.
- Scalability - They can be manufactured at a very small scale, allowing for high-density circuits.
One of the most promising applications of memristors is in computer memory, specifically in resistive random-access memory (ReRAM).
Memristors are also being used to develop neuromorphic chips that mimic the architecture and efficiency of the human brain.
Due to their non-linear characteristics, memristors are useful in complex signal processing tasks.
Memristors have garnered significant attention in the field of Artificial intelligence (AI) due to their unique properties and potential applications. Here are some key ways in which memristors are important to AI:
One of the most promising applications of memristors in AI is in neuromorphic computing, a subfield that aims to mimic the architecture and efficiency of biological neural networks. Memristors can be used to create artificial synapses, the connections between neurons, in neuromorphic chips. Their ability to "remember" previous states allows for energy-efficient learning algorithms, closely resembling the adaptive learning processes found in biological systems.
Traditional computing architectures consume significant amounts of energy to perform machine learning tasks. Memristors, with their low energy requirements and non-volatile memory, offer a more energy-efficient alternative. This is particularly important for edge computing applications where power efficiency is crucial.
Memristors enable in-memory computing, where computation is performed directly within memory units rather than transferring data between the processor and memory. This reduces data movement, thereby increasing computational speed and reducing energy consumption. Such capabilities are highly beneficial for AI algorithms that require real-time processing and decision-making.
The small size and high density of memristor-based circuits make them highly scalable, an essential feature for complex AI algorithms and big data applications. Memristors can be integrated into existing semiconductor technologies, making them a practical choice for future AI hardware.
Memristors can serve as hardware accelerators for specific AI tasks, such as pattern recognition and natural language processing. By offloading these tasks from the central processing unit (CPU) to specialized memristor-based hardware, overall system performance and efficiency can be improved.
Memristors have the potential to revolutionize AI by offering energy-efficient, scalable, and high-performance computing solutions. Their unique characteristics make them well-suited for a range of AI applications, from neuromorphic computing to hardware acceleration.
See also: