Statistical classification
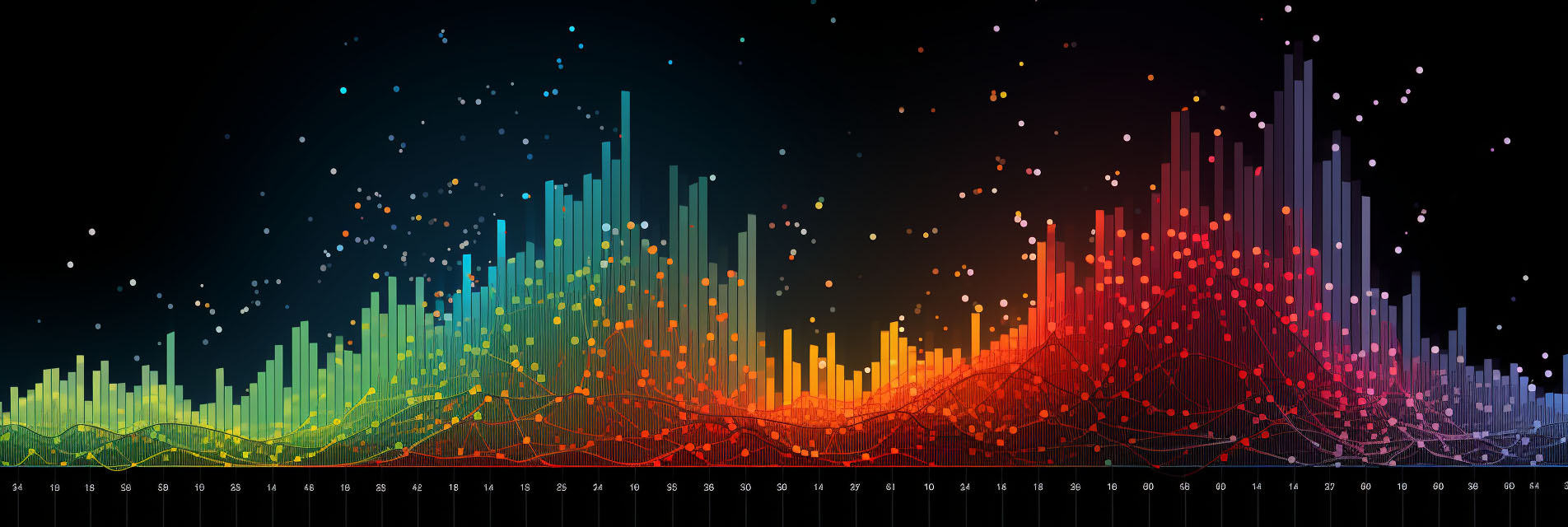
Statistical classification refers to supervised machine learning techniques for categorizing data points into classes. Key aspects:
- Algorithms learn from training data containing class labels.
- A classification model is built to predict the class of new unlabeled points.
- Probability theory determines class membership based on likelihood.
- Useful for predicting categorical variables in data.
Major approaches include:
- Logistic regression for binary classification.
- Linear discriminant analysis finds separations between classes.
- Naive Bayes classifiers apply Bayesian probability.
- Support vector machines find optimal decision boundaries.
- Decision trees partition data points using tree-like models.
Classification is used for predictive modeling tasks like spam detection, disease diagnosis, sentiment analysis, and more.
Performance metrics include accuracy, precision, recall, and F1-score. Challenges include class imbalance, overfitting, and defining optimal features.
Classification provides fundamental machine learning tools for modeling and analyzing categorical data. Advances in deep learning have boosted classification capabilities.
See also: