Text-to-image diffusion model
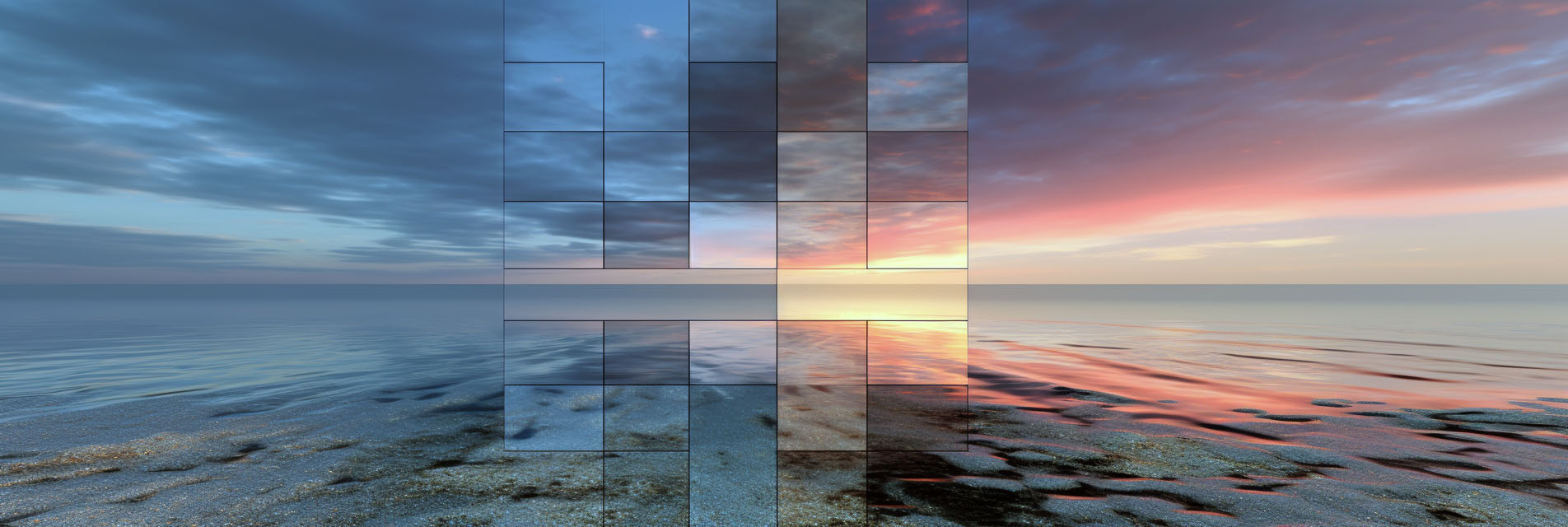
Text-to-image diffusion models are a class of generative deep learning models that can create photorealistic images from textual descriptions. They have emerged as a leading approach for controllable image generation using natural language.
Text-to-image generation is posed as a sequential refinement process. It starts with a noise image and improves it iteratively based on the text prompt. Noise is added after each refinement step allowing exploration of the image space. Diffusion models are trained to reverse this noise injection through hundreds of denoising steps.
At each step, the model conditions on the text embedding and current image to predict how to denoise and sharpen the image. After complete denoising, the output is a high-fidelity image reflecting the text. Transformer architectures encode the text into a latent representation that guides the image generation process.
DALL-E and Imagen from OpenAI as well as Google's Parti are popular examples built using variational autoencoders with diffusion for text-conditional image synthesis. They can generate creative visuals like "an armchair in the shape of an avocado" from scratch.
Text-to-image diffusion models allow granular control over image attributes like style, composition, and objects through natural language prompts. Pre-training on large datasets enables them to handle a diverse range of concepts and contexts. The generated images showcase photorealism and coherence not achieved by previous text-to-image GAN methods.
Overall, text-to-image diffusion drives progress in controllable generative modeling and multimodal AI.