Text mining
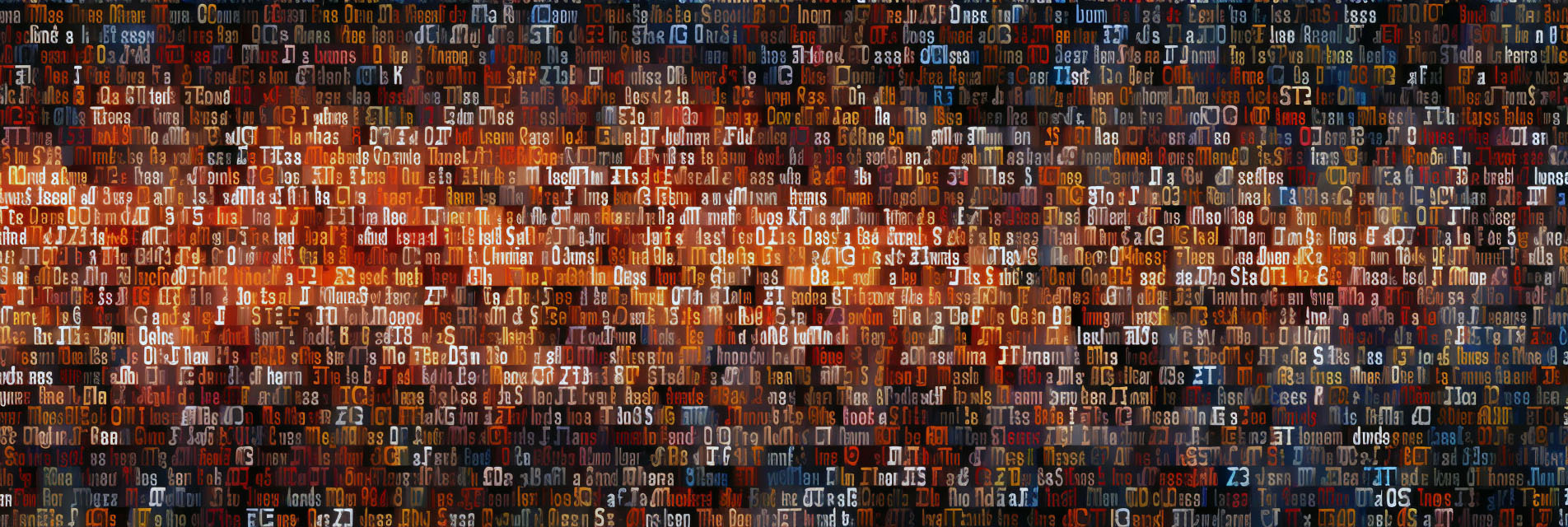
Text mining is the process of deriving high-quality information from text data through the identification of patterns and trends. It involves the application of natural language processing, machine learning and analytical methods to extract and classify patterns, trends, topics, sentiments, and other useful insights from unstructured text data. It is a multidisciplinary field that uses techniques from natural language processing, machine learning, and statistics. The key aspects of text mining include:
- Text preprocessing - Transforming text into a suitable format for mining by cleaning, normalizing, parsing, and transforming text.
- Information retrieval - Finding relevant documents, passages, or words from a text corpus. This uses search and information extraction techniques.
- Information extraction - Identifying key phrases, relationships, or facts contained in text and structuring into a database.
- Topic modeling - Discovering main topics or themes that pervade a collection of documents. Algorithms like LDA are used.
- Sentiment analysis - Detecting subjective opinions, emotions, evaluations and attitudes behind text using NLP.
- Summarization - Generating a short, condensed version highlighting the key ideas from a longer text document.
- Classification - Assigning categories or labels to documents using supervised, semi-supervised or unsupervised machine learning algorithms.
Text mining has its roots in the field of data mining, which involves the extraction of useful information from large datasets. The advent of the internet and the exponential growth of textual data led to the emergence of text mining as a distinct field. Over the years, text mining has evolved to include a wide range of techniques and applications, from sentiment analysis to topic modeling and information retrieval.
The key applications of text mining include search, metadata tagging, customer relationship management, business intelligence and predictive analytics. It enables businesses to uncover insights from customer feedback, social media, surveys, news, reviews and internal documents.
See also:
References:
- Feldman, Ronen; Sanger, James (2007). "The Text Mining Handbook: Advanced Approaches in Analyzing Unstructured Data". Cambridge University Press.
- Aggarwal, Charu C.; Zhai, ChengXiang (2012). "Mining Text Data". Springer.